A group of Canadian health care specialists, researchers and technologists is using Wi-Fi-based motion sensor data to detect depression in older adults.
The research, conducted at McGill University and the Mila-Quebec AI Institute, looked into how artificial intelligence can be used to accurately detect depression among older participants without using intrusive wearable devices.
It’s called HOPE, for Home-Based Older Adults’ Depression Prediction. And while the development and testing of this new machine learning model is a big step forward, seniors’ tech already represents a huge sector of products and services designed to support our growing population of older adults.
Mental health concerns and symptoms of depression among older adults is a growing public health concern, and the HOPE team cites studies estimating that up to 20 per cent of older adults living in the community experience some form of depression; in long-term care facilities, it can be 30 to 40 per cent.
Despite such high figures, researchers say nearly half of depression cases are never diagnosed, leading to more detrimental effects on physical and emotional health, increased hospitalization rates, and a reduced quality of life for our senior citizens.
So principal investigator Professor Samira A. Rhaimi led a team from McGill University and Mila-Quebec AI Institute to see if the everyday movement and sleep patterns of older adults, watched digitally by Wi-Fi-based data sensors, could provide early indicators of depression.
Traditional detection methods, often based on in-person clinical interviews and the data gathered from wearable-based monitors on the wrist or around the neck, smart devices placed in the home, or even a network of video cameras in an elder care facility, can be resource-intensive and somewhat intrusive or inconvenient, particularly for older adults who may face concerns adapting to technology.
The HOPE model addresses such challenges by using existing Wi-Fi infrastructure to allow for a continuous passive monitoring of the environment and its occupants without requiring any active participation from users.
It’s a process dubbed Wi-Fi Sensing by some.
But with a cited accuracy rate nearing 90 per cent, this hopeful new solution to non-intrusive mental health monitoring offers people a chance at early intervention.
“Too often, the mental health of older adults is overlooked, leaving many to suffer in silence without the care and attention they deserve,” Professor Rahimi said when the research was published. “Our HOPE model could act as a caring friend who looks out for signs of depression in older adults using everyday Wi-Fi data to spot potential issues early on and without being intrusive. It’s about using technology to lend a helping hand, especially for those who might find it hard to reach out themselves.”
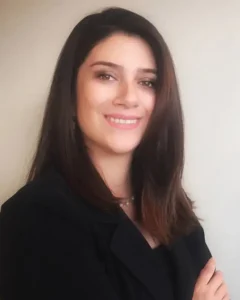
Samira Abbasgholizadeh-Rahimi, McGill University and Mila-Quebec AI Institute.
Samira Abbasgholizadeh-Rahimi, BEng, PhD, is Canada Research Chair (Tier II) in AI and Advanced Digital Primary Health Care and an Assistant Professor in the Department of Family Medicine at McGill University and Mila-Quebec AI Institute; she is also Co-Director, McGill’s Collaborative for AI and Society (McCAIS).
She researches the development and implementation of advanced digital health technologies, such as AI-enabled decision support tools, and her work has long had an emphasis on vulnerable populations, such as older adults.
An important aspect of the HOPE model is the integration of what’s known as explainable AI (XAI), seen as a way to boost both data transparency and clinical interpretability.
By keeping AI-driven predictions open and actionable, the HOPE model is designed to build trust and confidence among users while making possible even earlier detection of depression among older adults in the community, and Rahimi’s lab used explainable machine learning models to identify some key factors in depression detection, such as sleep habits.
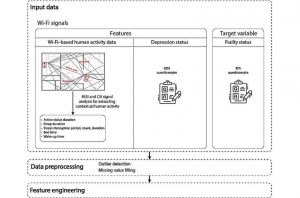
Research and analysis conducted as part of the HOPE project led to development of an AI tool that acts like “a caring friend”, one who uses everyday Wi-Fi data to look for signs of depression in older adults.
The analysis showed that the most influential sleep-related indicators of depression factors were sleep duration, the number and duration of sleep interruptions, and the level of frailty (based, interestingly enough, on a globally-recognized medical diagnostic scale called the Edmonton Frail Scale, first developed at the University of Alberta more than a quarter century ago).
Although the findings were encouraging, the test population participating in the HOPE research project was small, so larger studies are needed to provide further evidence for this particular approach.
Dr. Rahimi told WhatsYourTech that she is indeed planning to expand this line of research. “Our team is exploring several interconnected areas, including the role of AI in better understanding and supporting sleep, depression, and overall mental well-being in older adults. We’re also working on ways to adapt the current model for more personalized, scalable interventions that can be integrated into community (including in CHSLDs) and home-based care settings.”
The study demonstrated how Wi-Fi technology, sensor data and appropriate processing can be used for mental health assessments, and to support early intervention efforts while improving the quality of life for older adults at risk of depression.
As mentioned, Wi-Fi Sensing is a promising non-intrusive approach for data gathering, and what’s known as human activity recognition (HAR).
We have tons of Wi-Fi-enabled devices in our homes, workplaces, pockets, and hands. While machine learning tools and artificial intelligence programs can get meaningful information from all the wireless propagation patterns bouncing around us (be they line-of-sight or otherwise), the machine learning and AI training that goes on requires good data models and robust datasets about human activities, conditions, and behaviours.
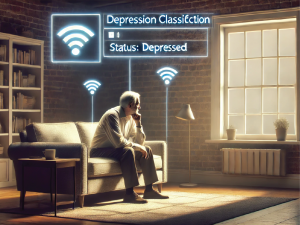
A report published in JMIR Aging looks into the the development and testing of a new AI model called HOPE, a tech-enabled platform that uses collected Wi-Fi data to help diagnose depression in older adults. The report, Development and Feasibility Study of HOPE Model for Prediction of Depression Among Older Adults Using Wi-Fi-based Motion Sensor Data: Machine Learning Study, was researched and written in Canada. © Shayan Nejadshamsi, Vania Karami, Negar Ghourchian, Narges Armanfard, Howard Bergman, Roland Grad, Machelle Wilchesky, Vladimir Khanassov, Isabelle Vedel, Samira Abbasgholizadeh Rahimi.
Various initiatives to enhance that capacity, such as the McGill/MILA project, are underway at any time, with datasets being built up to describe various human activities and actions, whether by individuals or multi-person groups.
They’re fuelling not only innovation in the tech sector, but hope among older adults battling depression, anxiety and other mental health challenges.
# # #
Free and confidential mental health information is available 24 hours a day, seven days a week in Ontario. Contact Mental Health Service Information Ontario toll free at 1 866 531-2600.
The Canadian Mental Health Association has information and resources as well; you can find them at https://cmha.ca/find-info/mental-health/general-info/
-30-